- source
- (Flageat, Cully 2020)
- tags
- ALife 2020, MAP-Elites, Quality diversity
Summary
MAP-Elites can be problematic in face of uncertainty because:
- individuals can be unexpectedly lucky
- the behavior space can be hard to estimate and result in misplacing individuals.
Some mitigation techniques have been explored, e.g in (Justesen et al. 2019) and this paper is about introducing another way of dealing with noisy domains without using sampling.
Here the main idea is to replace the MAP-elites grid by a “deep grid” with another dimension. This other dimension is used to store a population of individuals instead of a single individual for each elite. For each mutation, a single individual from the population is selected.
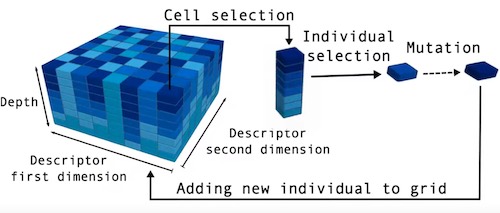
Figure 1: Slide from the Alife talk
Experiments show that deep grids are a data efficient extension of MAP-elites which enable reducing uncertainty on the behavior descriptors.
Bibliography
- Manon Flageat, Antoine Cully. . "Fast and Stable Map-elites in Noisy Domains Using Deep Grids". Artificial Life Conference Proceedings 32 (July). MIT Press:273–82. DOI.
- Niels Justesen, Sebastian Risi, Jean-Baptiste Mouret. . "Map-elites for Noisy Domains by Adaptive Sampling". In Proceedings of the Genetic and Evolutionary Computation Conference Companion, 121–22. GECCO '19. Prague, Czech Republic: Association for Computing Machinery. DOI.