- tags
- Neural networks
Implementation
Self-attention is a weighted average of all input elements from a sequence, with a weight proportional to a similarity score between representations. The input \(x \in \mathbb{R}^{L \times F}\) is projected by matrices \(W_Q \in \mathbb{R}^{F \times D}\), \(W_K \in \mathbb{R}^{F\times D}\) and \(W_V \in \mathbb{R}^{F\times M}\) to representations \(Q\) (queries), \(K\) (keys) and \(V\) (values).
\[ Q = xW_Q\] \[ K = xW_K\] \[ V = xW_V\]
Output for all positions in a sequence \(x\), is written
\[A(x) = V’ = \text{softmax}\left( \dfrac{QK^{T}}{\sqrt{D}} \right) V.\]
The softmax is applied row-wise in the equation above.
Possible interpretation
Keys and queries have a relatively simple interpretation. The keys are embeddings of tokens that expose some useful information about them:
The key \(K_3\) associated with cat
should probably encode some information about the fact that it’s a noun, that it refers to a living entity, an animal, etc. On the other hand, the key \(K_2\) encodes the fact that pretty
is an adjective, and is used to denote some positive things about the subject’s appearance. That key is probably close to keys for beautiful
and nice
.
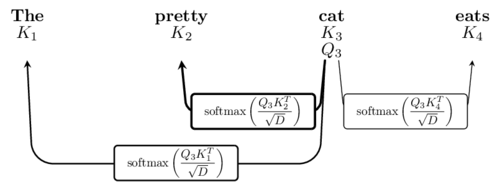
The query encodes another type of information about what types of keys would be useful for that particular token. In the case of query \(Q_3\) it is probably useful to attend to any adjective-like key that could show something interesting about the current word. Therefore, the quantity \(\text{softmax}\left( \dfrac{QK^{T}}{\sqrt{D}} \right)\) will be larger and will contribute more heavily in the resulting vector \(V’\). This is illustrated in the graph above with heavier edges.
Is it necessary?
Maybe not (Zhai et al. 2021).
Bibliography
- Shuangfei Zhai, Walter Talbott, Nitish Srivastava, Chen Huang, Hanlin Goh, Ruixiang Zhang, Josh Susskind. . "An Attention Free Transformer". Arxiv:2105.14103 [cs]. http://arxiv.org/abs/2105.14103.