- source
- (Grbic, Risi 2020)
- tags
- Meta-learning, Reinforcement learning, ALife 2020
Summary
In RL an important goal is to find agents that can quickly adapt to changing environments while avoiding unsafe states. However, in deep RL, there is often noise added to explore the action space: this can lead to unsafe part of the state-action space.
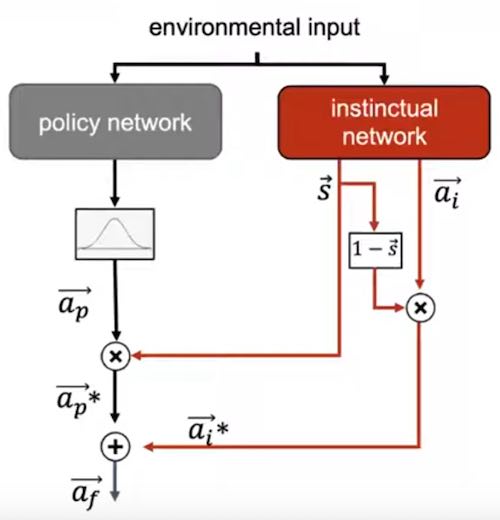
Figure 1: Slide from the Alife talk
The meta-learning setting of MAML is adapted to RL, with a policy network learning the policy in a standard way and a “instinctual network” which is fixed for a group of tasks and modulates the regular policy with its own action vector.
They show results on a navigation tasks with and without hazards and with and without instincts. It seems that instincts makes convergence slower when there are no hazards in the navigation task but allows to reach better fitness in the presence of hazards.
Bibliography
- Djordje Grbic, Sebastian Risi. . "Safe Reinforcement Learning Through Meta-learned Instincts". Arxiv:2005.03233 [cs]. http://arxiv.org/abs/2005.03233.