- tags
- Applied maths
Definition
The KL divergence is not symmetric. For \(P, Q\) defined on the same probability space \(\mathcal{X}\), KL of \(Q\) from \(P\) is \[ KL(P, Q) = \sum_{x \in \mathcal{X}} P(x) \log\left( \frac{P(x)}{Q(x)} \right) \]
It has two main interpretations:
- It is the information gain from using the right probability distribution \(P\) instead of \(Q\) or the amount of information lost by approximating \(P\) with \(Q\).
- The average difference in code length for a sequence following \(P\) and using a code optimized for \(Q\) to encode it.
Forward and reverse KL
Eric Jang’s blog has an interesting visual explanation of the difference between forward and backward KL.
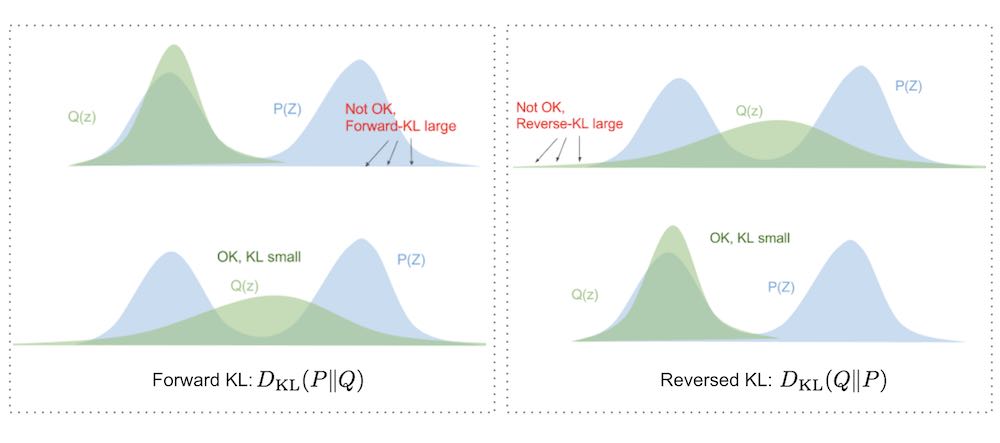
Figure 1: Difference between forward and backward KL
So in summary, minimizing forward-KL “stretches” your variational distribution \(Q(Z)\) to cover over the entire \(P(Z)\) like a tarp, while minimizing reverse-KL “squeezes” the \(Q(Z)\) under \(P(Z)\).